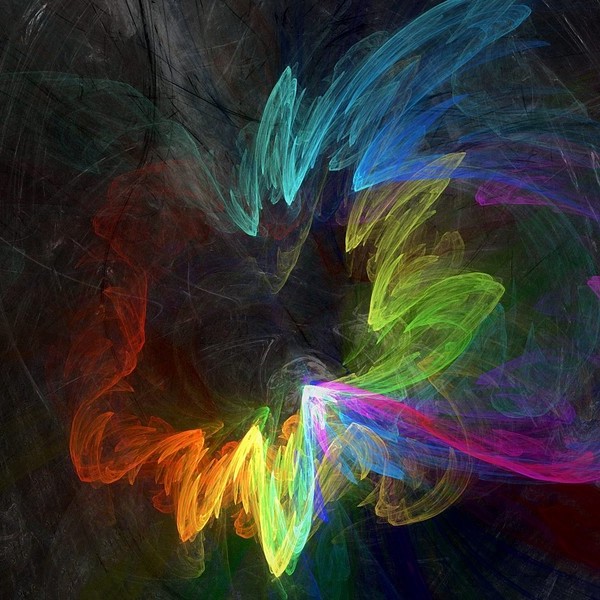
Of course anyone can vote for who they like, or not vote at all, no one’s saying otherwise. It’s Harris’s job to earn your vote, and she clearly hasn’t. But pushing third parties as the solution to any problem is going to do more harm than good until we get a better election system. It may feel better to vote for a party that more clearly aligns with your positions, but if they have no path to actually acquiring any power to make change, you’re doing nothing while feeling like you did something. Changing the policies of a flawed party that actually has power is much harder, and yes, there might be compromise or half-measures, but that’s an infinitely more productive path. (More productive than that is doing direct action outside of the electoral system entirely, but both things can be done at the same time.)
The UN is supposed to be a toothless, executively dysfunctional institution, that’s a feature, not a bug. Its members are nations, whose entire purpose is to govern their regions of the planet. If the UN itself had the power to make nations do things, it wouldn’t be the United Nations, it’d be the One World Government, and its most powerful members absolutely do not want it to be that, so it isn’t.
It’s supposed to be an idealized, nonviolent representation of geopolitics that is always available to nations as a venue for civilized diplomacy. That’s why nuclear powers were given veto power: they effectively have veto power over the question of “should the human race continue existing” and the veto is basically a reflection of that. We want issues to get hashed out with words in the UN if possible, rather than in real life with weapons, and that means it must concede to the power dynamics that exist in real life. The good nations and the bad nations alike have to feel like they get as much control as they deserve, otherwise they take their balls and go home.
It’s frustrating to see the US or Russia or China vetoing perfectly good resolutions and everyone else just kind of going “eh, what can you do, they have vetoes,” but think through the alternative: everyone has enough and decides “no more veto powers.” The UN starts passing all the good resolutions. But the UN only has the power that member nations give it, so enforcement would have to mean some nations trying to impose their will on the ones that would’ve vetoed. Now we’ve traded bad vetoes in the UN for real-world conflict instead.
What that “get rid of the vetoes so the UN can get things done” impulse is actually driving at is “we should have a one world government that does good things,” which, yeah, that’d be great, but it’s obviously not happening any time soon. Both articles mention issues and reforms that are worthy of consideration, but the fundamental structure of the UN is always going to reflect the flaws of the world because it’s supposed to do that.